December 1, 2023
2 min read
Scientists can finally predict a chemical’s odor without having a human sniff it
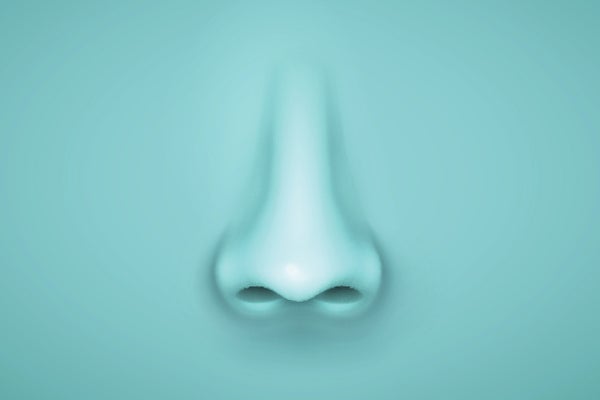
To a human nose, hydrogen sulfide smells like rotten eggs, geranyl acetate like roses. But the problem of guessing how a new chemical will smell without having someone sniff it has long stumped food scientists, perfumers and neuroscientists alike.
Now, in a study published in Science, researchers describe a machine-learning model that does this job. The model, called the Principal Odor Map, predicted smells for 500,000 molecules that have never been synthesized—a task that would take a human 70 years. “Our bandwidth for profiling molecules is orders of magnitude faster,” says Michigan State University food scientist Emily Mayhew, who co-led the study.
.jpg?w=1000)
The color of light is defined by its wavelength, but there’s no such simple relationship between a molecule’s physical properties and its smell. A tiny structural tweak can drastically alter a molecule’s odor; conversely, chemicals can smell similar even with different molecular structures. Earlier machine-learning models found associations between the chemical properties of known odorants (called chemoinformatics) and their smells, but predictive performance was limited.
In the new study, the researchers trained a neural network with 5,000 known odorants to emphasize 256 chemical features according to how much they affect a molecule’s odor. Rather than using standard chemoinformatics, “they built their own,” says Pablo Meyer Rojas, a computational biologist at IBM Research, who was not involved in the study. “They directly inferred the properties that are related to smell,” he says—although how the model arrives at these predictions is too complex for a human to understand.
The model creates a giant map of odors, with each molecule’s coordinates determined by its chemical properties. The model also predicts how each molecule will smell to a human, using 55 descriptive labels such as “grassy” or “woody.” Remarkably, similar-smelling odorants appeared in clusters on the map—a feature prior odor maps couldn’t achieve.
The team then compared the model’s scent predictions with the judgments of 15 humans trained to describe new odorants. The model’s predictions were as close as those of any human judge to the panel’s average descriptions of the new scents. It could also predict an odor’s intensity and how similar two molecules would smell—two things it was not explicitly designed to do. “That was a really cool surprise,” Mayhew says.
The model’s main limitation is that it can predict the odors of only single molecules; in the real world of perfumes and stinky trash bags, smells are almost always olfactory medleys. “Mixture perception is the next frontier,” Mayhew says. The vast number of possible combinations makes predicting mixtures exponentially more difficult, but “the first step is understanding what each molecule smells like,” Meyer Rojas says.